1STAFF POWERED BY MICROSOFT MICROSOFT AI & 1STAFF COPILOT
My Journey with OpenAI in Daily Work
As someone intrigued by generative AI from the outset, I quickly became an early adopter in November 2023 when I signed up for OpenAI’s Chat GPT. This marked the start of an exploration into how AI could augment and improve my daily tasks, including drafting emails, blogs, proposals, product documents, and statements of work.
Initially, my interest was piqued by the potential for basic improvements in textual content. And to be clear, I am not a mathematician or data scientist and I am in no way delving into how this thing actually works. What I do understand fundamentally is that it is a numbers-and-probability based algorithm, Very clever for sure, but just crunching lots of numbers.
The Evolution of AI in Knowledge Work
Over the past nine months, I’ve experimented with a variety of AI providers, including Chat GPT, Gemini, Llama, Perplexity and Microsoft Bing (now Microsoft Copilot). Each offered various capabilities and insights, and through these experiences, I’ve identified three distinct use cases for generative AI in knowledge work. Note that I’m not the creative type and I haven’t spent much time in the AI imaging space.
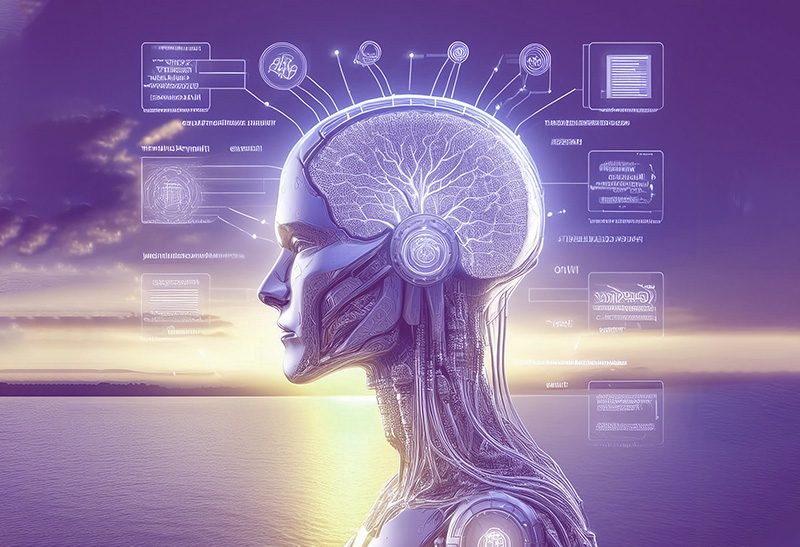
"Becoming an early adopter of OpenAI's Chat GPT marked the start of my exploration into how AI could enhance my daily tasks."
Initial basic usage: Bring Your Own AI
The first use case is straightforward and likely the most common: leveraging generative AI for individual tasks. This often involves sitting at my desk, drafting an email or other text block, and using an AI tool like ChatGPT or Microsoft Copilot to create a first draft. Sometimes, I start with a draft and use the AI to refine it, making it more formal or friendly, shorter or longer, structured in bullet points or data in tables. This process typically involves copying and pasting content between Word and Outlook and the AI interface, iterating until I am happy with the output.
However, this approach comes with a significant organizational risk. When employees use different AI engines and prompt styles and directions, consistency is a challenge. The person sitting beside me will likely end up with a different tone, tenor and style in their text than I do, or indeed everyone else. So, communications from the company become stylistically varied.
Moreover, thoughtless casual copying and pasting data from internal systems into public AI engines can inadvertently expose confidential information, posing a potential privacy breach and security threat.
Evolving usage: Integrated AI within Packaged Applications
The second use case involves AI being seamlessly integrated into the packaged applications I use daily. I see this integration in email and CRM systems, help desk systems, financial management tools, and of course right the way across the Microsoft Dynamics 365 platform. Unlike the first use case, where the user provides context to the AI, integrated AI automatically devises context from the system and suggests ways to assist based on my current data views and system activities. For instance, it might predict what I am trying to do and offer to automate it, or it might suggest relevant tasks, reports, or analyses.
This context-aware capability is where many organizations will see the most significant personal productivity gains. It allows systems to interrelate data points automatically, proposing actions, extractions, analyses, viewpoints, or even predictions.
A key point for me in this use case is “human in the loop.” AI is suggesting, drafting, surmising. But the final decision on using the AI output or doing the AI suggested activity is usually best left in the hands of a human to assess relevance, accuracy, bias, tone, timing and if the consumer of the output is another human, the all-important empathy.
Enterprises are likely to be more comfortable with this setup since the large language model (LLM) is typically dedicated, secure, has built-in security controls to protect sensitive information and may well be domain/industry/role specific.
An example of this is our 1Staff Copilot offering which gives salespeople and recruiters using our 1Staff Front Office CRM and applicant tracking system a virtual assistant for a wide range of tasks safely within the corporate philosophy and security guardrails.
Corporate level expansion: Building Custom AI Models
The third and most exciting use case for me is the ability to build custom AI models. Within a corporately approved and controlled AI environment, such as Microsoft Azure and Copilot, I can use tools like Copilot Studio to create and train focused and contextually relevant knowledge stores. These custom models can then be interrogated to respond to specific queries.
One practical application I’ve explored is using a custom Copilot model to streamline responses for requests for proposals (RFPs). By feeding past RFP responses into an RFP response bot, I can speed up crafting responses for new RFPs. The bot can draw from previous answers and our documented policies and procedures, providing substantiated responses that align with our standards.
The challenge I see for companies investing in this area is the collation, review and stewardship of the volume of training documents. Many companies do not have an adequately structured, cohesive, domain-siloed document store sufficient to train an LLM rigorously enough to deliver worthwhile and reliable output.
I read a blog elsewhere recently suggesting most companies don’t actually have much internal documentation worth retrieving – the blunt message was, and I quote, “Fix. Your. S$!t.”!
Challenges and lessons Learned.
Most people by now will have heard about and possibly experienced hallucinations – where the LLM model frankly just makes stuff up. It hasn’t been a concern in my personal experience but I’m not typically stretching far outside an average knowledge worker’s remit. I also learnt about the “temperature” setting for prompts early on and I always set that low for business purposes. The other amusing thing is that LLM’s can’t seem to count. Generally, I am not seeking that function, but it is something to be wary about for use cases involving numerical data.
DISCLOSURE: I used AI to write this blog. Of course I did! I recorded myself in Microsoft Teams rambling on about this topic for 5 or 6 minutes and saved it as a transcript. As an experiment, I then pasted that unedited text, umms and ahhs and all, separately into Microsoft Copilot, Open AI and Gemini with a vanilla “make this into a blog” prompt for each.
Being the mandatory “human in the loop” I then reviewed each output and took bits that I liked from all three along with my own editing to build the semi-final output. I then pasted that back into Open AI prompting for a common conversational style and casual tone with a temperature of 0.2. I had a couple of attempts at that but frankly I didn’t like the output, so the final version above is substantially “my own work” and when I put it through Quillbot’s free AI detector I got a “100% human” rating.
So, what was the point of using AI in the first place I hear you ask. It gets me started. It structures my rambled thinking. It collates and orders the key points. It gives me a draft to work with. And it gets me to do it. That’s sometimes the hardest part – getting something done, from scratch.
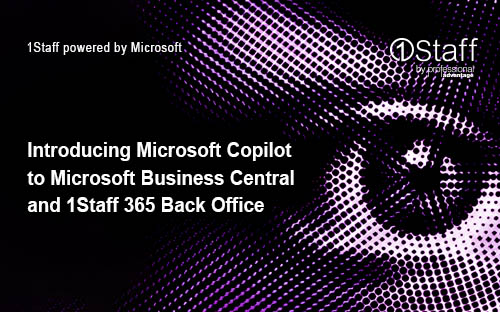
More from the blog!
Introducing Microsoft Copilot to Microsoft Business Central and 1Staff 365 Back Office
We are excited to announce the introduction of Microsoft Copilot features to Microsoft Business Central and 1Staff 365 Back Office.
Read the blog...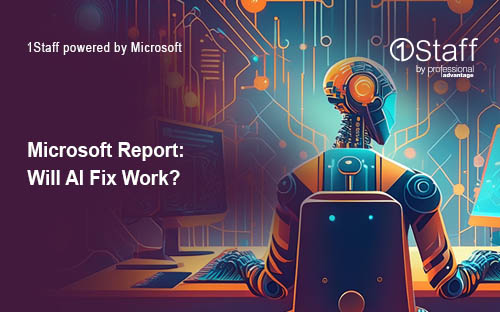
Read the full Microsoft Work Trend Index Report
Will AI Fix Work?
The pace of work is outpacing our ability to keep up. AI is poised to create a whole new way of working.
Read more...